MARVEL labs develop a machine learning model for the electron density
by Fiorien Bonthuis, NCCR MARVEL
When a scientist encounters a new molecule and sets out to study its properties, one of the first questions they’re likely to ask is: where are the electrons? It’s an important question, because the types of bonds atoms and molecules can form crucially depends on the number and positions of their electrons: covalent bonds require shared electrons, ionic bonds involve exchanged electrons, and so on. Tracking the positions of the electrons and the concomitant forces, we get a simple model of how atoms form molecules. Except, of course, things are never that simple.
People often talk about the structure of the atom as if it’s a tiny version of our solar system: the electrons travel around the nucleus like the planets orbiting the sun (with electrostatic rather than gravitational interactions holding things together). This picture makes it look as if we can determine the positions of the electrons exactly, just like we can calculate the trajectories of the planets. However, the classical physics that allows us to predict the planetary motions breaks down at the atomic scale, where quantum physics rules supreme. In fact, classical physics predicts that the atom would collapse, with the electrons crashing into the nucleus. Quantum physics explains why this doesn’t happen. The price we pay is uncertainty: in a quantum world, we can never know exactly where the electrons are.
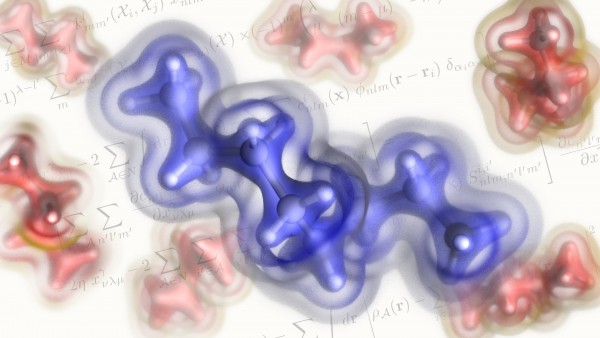
Instead, what we have is the probability of finding an electron at a certain point in the space around the nucleus of an atom. The electron density is a measure of this probability. A map of the electron density shows us where electrons are likely to be, as well as how negative charge is distributed. As such, the electron density can be used to identify covalent bonding patterns and non-covalent interactions, and to determine the geometry of molecules.
Unlike the wave-function, which fully describes the state of the quantum mechanical system but which is not itself a physical observable, the electron density can be measured experimentally, using electron diffraction and x-ray crystallography. At the same time, the electron density can be used as the basic variable in first-principles calculations: the Hohenberg-Kohn theorem tells us that there is a one-to-one correspondence between the electron density and the solution of the Schrödinger equation. The upshot of this is that a system’s ground-state properties can be expressed as functionals of the electron density. Density Functional Theory, the most commonly used framework in computational chemistry, is based on this insight.
“Electron density is used absolutely everywhere in quantum chemistry. But computing the electron density from the positions of the atoms in a molecule requires significant effort,” Clemence Corminboeuf says. “A machine learning method would allow us to sidestep these calculations and access the ground-state electron density for a given configuration directly. This would have broad implications, including real-time visualisation of chemical fingerprints, acceleration of DFT calculations, and exact treatment of the electrostatic interactions in atomistic simulations. It would also be of use in the analysis and interpretation of experimental techniques for probing the electron density, such as transmission electron microscopy and x-ray crystallography.”
Developing an effective machine learning technique for the electron density is not trivial however. “An important drawback of existing models, such as the landmark model developed by Brockherde et al. (Nat. Comm. 2017, 8, 872), is that they are not easily transferable to large and flexible systems,” Michele Ceriotti says. “This limitation is intrinsic to the structure of the model, which learns the density of molecules as a whole. As a result, it is effectively limited to interpolating between known, complete structures of relatively rigid, small molecules.”
The MARVEL team decided to try a more Lego-like approach. “Our idea was to construct a model such that the densities of larger and more flexible molecules can be learned from the densities of smaller units,” Ceriotti says. To achieve this, the authors used an atom-centred, localised basis set to represent the charge density in a way that is reminiscent of the local expansions of the wave-function, in combination with a symmetry-adapted regression scheme that is based only on knowledge of the positions of the nuclei with the total electron density as the target property. “Less technically, what that means is that our model determines the most convenient way to decompose the charge densities of molecules into atom-centred contributions. This effectively creates chunks of electron cloud centred around a small-ish number of atoms. It can then put these chunks together and rotate them as necessary to predict the densities of new, larger molecules.” Ceriotti explains.
The authors tested their model on hydrocarbon molecules of increasing complexity. First, using a training set of hydrocarbons with 2 and 4 C atoms, they show that their model accurately predicts the densities of new C2 and C4 hydrocarbon configurations, achieving an error of the order of 1%. Given that the reconstructed density is based exclusively on the nuclear position, this could be used for assisting the analysis of x-ray experiments, the authors suggest. Second, using a training set with C4 hydrocarbons, the authors show that the model accurately predicts the electronic charge of larger, C8 counterparts.
“This latter result really demonstrates that our atom-centred, symmetry-adapted approach works to achieve transferability, using a training set of smaller molecules to predict the densities of larger ones,” Corminboeuf says. “In fact, as we state in the paper, this work can be seen as the first, successful attempt to apply machine learning in a transferable way to molecular properties that cannot be simply decomposed as the sum of atom-centred values, but exhibit a richer, more complex geometric structure. As such, the framework we have introduced could be a valuable starting point for machine learning approaches to other complex properties, such as the Hamiltonian, the density matrix, vector fields, density response functions, et cetera.”
Combining Ceriotti’s and Corminboeuf’s expertise in machine learning and in quantum chemistry, respectively, the work is also a nice demonstration of the kind of collaboration the NCCR MARVEL fosters. “Frankly, we would probably have collaborated anyway,” Ceriotti says “But the MARVEL framework provides a clear common goal, making collaboration both easier and more effective.” “Collaborations like these are really beneficial for our students too,” Corminboeuf adds. “It allows them to work with and learn from people with a very different expertise. The exposure to a different field is very valuable and it really broadens their horizon.”
Reference: ACS Cent. Sci. 2019, 5, 1, 57-64
Transferable Machine-Learning Model of the Electron Density
Andrea Grisafi†§, Alberto Fabrizio‡§ , Benjamin Meyer‡§, David M. Wilkins†, Clemence Corminboeuf‡§ , and Michele Ceriotti*†
† Laboratory of Computational Science and Modeling, IMX, École Polytechnique Fédérale de Lausanne, 1015 Lausanne, Switzerland
‡ Laboratory for Computational Molecular Design, Institute of Chemical Sciences and Engineering, École Polytechnique Fédérale de Lausanne, CH-1015 Lausanne, Switzerland
§ National Centre for Computational Design and Discovery of Novel Materials (MARVEL), École Polytechnique Fédérale de Lausanne, 1015 Lausanne, Switzerland
*E-mail: michele.ceriotti@epfl.ch
ACS Cent. Sci., 2019, 5 (1), pp 57–64
DOI: 10.1021/acscentsci.8b00551
Publication Date (Web): December 26, 2018
Low-volume newsletters, targeted to the scientific and industrial communities.
Subscribe to our newsletter