New machine learning approach enables accurate determination of Hubbard parameters at virtually no cost
By Nicola Nosengo/NCCR MARVEL
Machine learning is finding more and more applications in scientific research, and computational material science is no exception. At the end of the day, its goal is to make very complex calculations – such as the use of quantum mechanics to describe the electronic structure of materials - tractable. Machine learning offers a way to “learn” some values that are critical for these computations without having to calculate them entirely from first principles.
A new article in npj Computational Materials, by members of Nicola Marzari’s MARVEL laboratories at EPFL and at the Paul Scherrer Institute, shows how machine learning can reduce the time and computational cost of a widely used material simulation method, namely density-functional theory with extended Hubbard functionals (DFT + U + V).
Standard DFT – the cornerstone of computational materials science – has shortcomings when it is applied to complex materials containing transition-metal or rare-earth elements. The addition of Hubbard corrections substantially alleviates the problem. These corrections rely on two parameters: an on-site U which localizes electrons around an atom, and an inter-site V which balances U by reinforcing interatomic orbital hybridization. But how to find the right U and V values? This can be done empirically, if one has enough prior knowledge (i.e. experimental data) of the system, or through first-principles calculations which are though computationally quite expensive.
To achieve a better balance between accuracy and computational cost in calculating Hubbard U and V parameters, the team has turned to a recently developed class of neural networks called “equivariant neural networks”, that rely on specific symmetries between the input and output in their mathematical function.
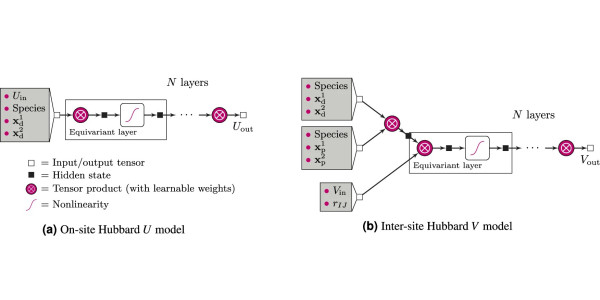
(a) The model for predicting on-site U values, (b) the model for inter site V values. Uin and Vin are the input Hubbard parameters, while Uout and Vout are the outputs. [Uhrin et al, npj Comput Mater (2025)].
“A lot of people who are using machine learning nowadays start from the atomistic structure, that is the position of chemical elements in the crystal structure”, explains the study’s first author Martin Uhrin. “But the values we want to predict here are fundamentally about electrons, so it makes sense to have a machine-learning model that starts from what the electrons are doing, rather than the ions. This new class of machine learning models makes it very easy. We use the atomic occupation matrix, that tells you where the valence electrons are around the transition metals, as a direct input to the learning model”.
In fact, the method implies training two separate models – one for the U parameter and one for the V – to work independently of one another. To train them, the researchers created a dataset using available data on 12 materials spanning various crystal structures and compositions, belonging to the classes of battery materials, rare earth nickelates, and other materials. “But the model is very general and can be used for any class of materials provided it was trained on a large and diverse dataset” says co-author Luca Binci.
As machine learning trainings go, this one was not particularly long or computationally heavy. “Training the two models for U and V was in the end far cheaper than calculating the Hubbard parameters directly with first-principles methods for a single configuration of a single material” says co-author Austin Zadoks.
The trained models performed very well in calculating both the U and V parameters themselves, as well as some downstream properties such as magnetic moments or voltages. For these properties, in particular, the error margins are below 1 per cent. “This shows that you can have the cake and eat it”, says Uhrin, “You can replace parts of the calculation with machine learning and keep the overall accuracy, which would allow the method to be used in high-throughput screening studies, for example looking at thousands of materials to find the better battery candidates”.
"The model weights and data are already public and available for users", says co-author Iurii Timrov, "but now the focus is on training models on a much larger set of elements and materials". The result will be made publicly available to the community at large through the Materials Cloud, the open-source infrastructure for computational materials science developed within NCCR MARVEL. “Scientists will be able to take their material of interest and use the model to predict the Hubbard parameters almost for free, thus bypassing very expensive first-principles calculations”, explains Timrov.
Reference
Martin Uhrin, Austin Zadoks, Luca Binci, Nicola Marzari and Iurii Timrov, Machine learning Hubbard parameters with equivariant neural networks. npj Comput Mater 11, 19 (2025). https://doi.org/10.1038/s41524-024-01501-5.
Low-volume newsletters, targeted to the scientific and industrial communities.
Subscribe to our newsletter