New Machine Learning Approach Speeds Investigation of Chemical Shifts in Molecular Solids
by Carey Sargent, EPFL, NCCR MARVEL
A four orders of magnitude speed up is a real game changer, making it possible to apply NMR crystallography to new classes of compounds. And this is with a rough implementation of the machine learning scheme – we expect to be able to make ShiftML more general, accurate and at least 10x faster.
— Michele Ceriotti, NCCR MARVEL member
The trained model was able to correctly determine the structures of cocaine and the experimental drug AZD8329 and can easily be scaled up to very complex structures, including the largest known today.
The approach, known as ShiftML and described in a Nature Communications paper by scientists led by Professor Michele Ceriotti of the NCCR MARVEL member Laboratory of Computational Science and Modelling (COSMO) and Professor Lyndon Emsley, head of the Laboratory of Magnetic Resonance, allowed the calculation of chemical shifts for structures with ~100 atoms in less than one minute, reducing the related computational cost by a factor of as much as 10,000 compared to current density-functional theory chemical shift calculations. The model calculated the shifts of six of the largest structures known in less than six CPU minutes, compared with an estimated 16 CPU years using the equivalent DFT approach.
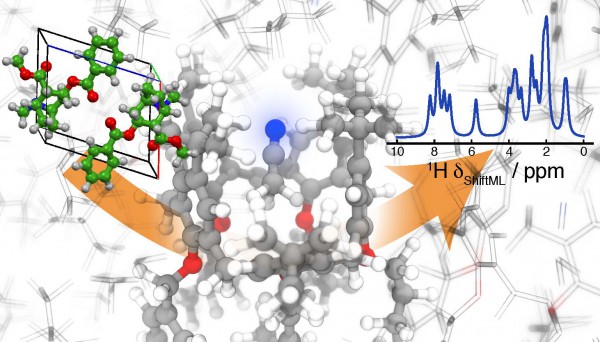
ShiftML will make it possible to apply NMR crystallography to complex supramolecular aggregates, which form crystals containing thousands of atoms in the periodic repeat unit. Photo: Albert Hofstetter
ShiftML may then help researchers in materials and pharmaceutical chemistry to determine the structures of molecular solids and identify variants—even for previously inaccessible molecules—much more rapidly and at lower computational cost than techniques that rely on electronic-structure calculations.
This is really exciting because the massive acceleration in computation times will allow us to cover much larger conformational spaces and correctly determine structures where it was previously just not possible.
—Lyndon Emsley, head of EPFL's Laboratory of Magnetic Resonance.
Recent advances in solid-state NMR have enabled the rapid development of chemical shift-based NMR crystallography, now widely used to determine molecular solid structures and validate known polymorphs. Recent studies suggest that this approach is at least comparable with traditional methods such as single crystal X-ray diffraction in terms of structural accuracy.
The problem, however, is that the computational cost is severely limiting: the structure determination relies on the comparison between measurements and reference calculations based on density-functional theory (DFT) electronic structure methods. The computational cost scales with the cube of the number of atoms and this prevents it from being applied to larger and more complex crystals. Using more accurate ab initio calculations, going beyond DFT, would make the expense prohibitive.
Machine learning has recently emerged as a way of overcoming the need for quantum chemical calculations. Many factors, including the lack of an experimental database of shifts, have nonetheless hampered the development of such methods for use in molecular solids. The EPFL scientists found a way around these problems by developing an ML framework that captures the local environments of individual atoms and is trained on DFT calculated chemical shifts for structures taken from the Cambridge Structural Database (CSD).
Though no experimental shifts were used in training, the model was nonetheless sufficiently accurate to correctly determine the structure of cocaine and the experimental drug AZD8329. The researchers also successfully calculated the chemical shifts of six structures with between 768 and 1,584 atoms in the unit-cells, showing that the model can calculate the chemical shifts of very large molecular crystals. Furthermore, the accuracy of the method does not depend on the size of the structure and the prediction time is linear in the number of atoms.
A web version of the model is publicly available at http://shiftml.epfl.ch.
The paper, Chemical Shifts in Molecular Solids by Machine Learning, can be found here.
DOI: 10.1038/s41467-018-06972-x
Low-volume newsletters, targeted to the scientific and industrial communities.
Subscribe to our newsletter