Machine learning approach predicts electron densities with DFT accuracy
By Carey Sargent, NCCR MARVEL, EPFL
Non-covalent interactions (NCI) govern a wide range of chemical phenomena and are essential to constructing molecular architectures. Evaluating them remains difficult though. One of the simplest and generally applicable methods involves using the total electron density ρ(r) as a starting point. It is generally obtained by solving the electronic structure problem with ab-initio computations. While accurate, such computations can become unwieldly if the variable has to be evaluated for thousands of different molecules or for very large systems such as peptides and proteins.
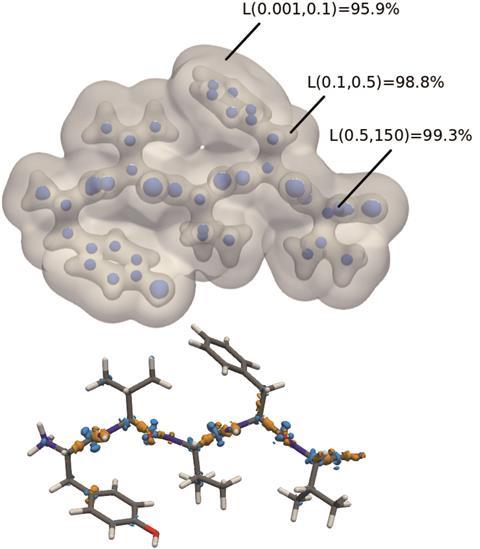
Photo credit: Clemence Corminboeuf/EPFL
Top: The predicted electron density of enkephalin. L represents the similarity at certain regions in space between the predicted density and that computed through DFT. Bottom: Major density differences between the predicted and computed electron densities. These are mainly along the peptide backbone, a feature not included in the dimer training sets
In the paper Electron density learning of non-covalent systems, NCCR MARVEL researchers presented a transferable and scalable machine-learning model capable of predicting the total electron density directly from the atomic coordinates. The approach is particularly well-suited to characterizing NCIs and was capable of handling complex chemical systems, predicting and analyzing the electron density of a collection of eight polypeptides of about 100 atoms at DFT accuracy in a few minutes. Such a robust method of analysis could help researchers understand complex interactions between biomolecules and potentially assist in the design of self-assembled materials and drugs.
The paper was covered in detail in an article published in Chemistry World.
Reference:
Electron density learning of non-covalent systems
Alberto Fabrizioab, Andrea Grisaficb, Benjamin Meyerab, Michele Ceriotticb and Clemence Corminboeuf
*ab
aLaboratory for Computational Molecular Design, Institute of Chemical Sciences and Engineering, École Polytechnique Fédérale de Lausanne, CH-1015 Lausanne, Switzerland.
bNational Centre for Computational Design and Discovery of Novel Materials (MARVEL), École Polytechnique Fédérale de Lausanne, 1015 Lausanne, Switzerland cLaboratory of Computational Science and Modeling, IMX, École Polytechnique Fédérale de Lausanne, 1015 Lausanne, Switzerland
Low-volume newsletters, targeted to the scientific and industrial communities.
Subscribe to our newsletter