Δ-learning HDNNP model shows promise in (QM)ML/MM MD simulations of condensed-phase systems
by Carey Sargent, NCCR MARVEL, EPFL
While classical fixed-charge force fields (FFs) can easily be used to perform molecular dynamics (MD) simulations of condensed-phase systems, quantum mechanics/molecular mechanics (QM/MM) MD simulations are a valuable alternative in cases where it’s necessary to model changes in the electronic structure.
In this approach, QM information is simulated with density functional theory (DFT) or through ab initio methods before being placed into the classical MM environment, allowing for the simulation of electronic structures of small systems in more realistic surroundings. The QM description forms a computational bottleneck, however, because it requires an expensive self-consistent field (SCF) procedure— an iterative method that optimizes the orbital coefficients of the wave function until convergence —and explicit treatment of all valence electrons. To speed up the process, semiempirical methods can be employed to describe the QM part, thereby trading accuracy against computational efficiency.
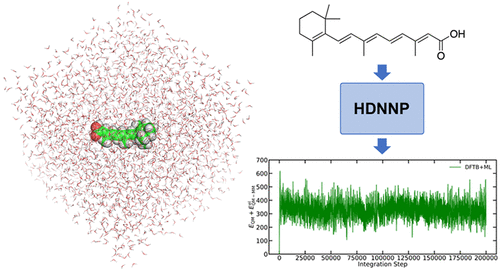
Retinoic acid in water as modelled by a machine learning approach that combines high-dimensional neural network potentials and Δ-learning
Applying machine-learned potentials in models trained on the potential-energy surface (PES) of QM systems is an alternative approach that has been increasingly investigated in recent years. This allows ML-MD simulations at a level of accuracy close to that of the electronic structure method used to generate the training set and drastically reduces the costs of the resulting ML-MD simulations compared to a normal DFT or ab initio MD simulation. Condensed-phase systems continue to pose a challenge for such approaches due to the abundance of important long-range interactions.
In the paper Machine Learning in QM/MM Molecular Dynamics Simulations of Condensed-Phase Systems, researchers led by Sereina Riniker, professor of Computational Chemistry at the Department of Chemistry and Applied Biosciences at ETH Zurich, set out to investigate ML models that simulate the QM region with an accuracy comparable to the reference QM method, while explicitly treating long-range interactions within a cutoff of 1.4 nm and allowing the QM region to be polarized by the MM particles. Standard QM/MM MD simulations at this level of accuracy are very expensive and only applicable to small systems.
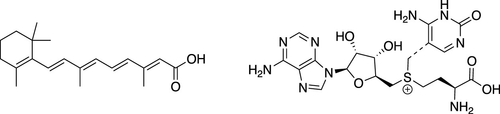
Two larger test systems used for MD simulations. (Left) Retinoic acid in water (50 QM atoms and about 2500 MM partial charges with a cutoff of 1.4 nm). (Right) Transition state of the chemical reaction between SAM and cytosine in water (63 QM atoms and about 3500 MM partial charges with a cutoff of 1.4 nm).
The researchers ultimately developed a workflow combining so-called high-dimensional neural network potentials (HDNNPs) and Δ-learning. HDNNPs encode the environment of each atom using symmetry functions that use Gaussians and trigonometric functions to map geometric information such as interatomic distances to higher dimensions. This high-dimensional description ultimately allows the HDNNP to learn the potential-energy surface accurately. The model can be trained on previously sampled data points and sampling can be done on a lower level of theory, as long as the trajectory samples all structures that are important for learning the PES. The data points are then recomputed on the desired level, and the HDNNP is trained to interpolate between the training data points.
Δ-learning involves training an ML model to reproduce the difference between an expensive, higher-level QM calculation and a cheaper, lower-level method. It reduces computational burden by allowing researchers to partially recover higher level output by actually performing the calculation with the less expensive method and then applying the trained ML model.
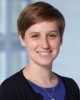
The approach described in the paper involved incorporating the MM environment as an element type in a HDNNP and engineering the fitted model to describe the PES such that the MM particles feel a force from the polarized QM particles, as desired. They tackled the problem of long-range interactions by choosing the semiempirical approach of density functional tight binding as the lower-level method in the Δ-learning scheme. The overall approach drastically reduces the complexity of generating the training set because not all atoms are treated on a QM level. It also allows for the use of a larger cut-off and therefore the inclusion of long-range interactions directly in the ML model.
The final model was ultimately tested through actual (QM)ML/MM MD simulations of the relatively large systems of retinoic acid in water (50 atoms in the QM zone and approximately 2500 classical partial charges) and the transition state of the chemical reaction between S-adenosylmethionate (SAM) with cytosin (63 atoms in the QM zone and approximately 3500 partial charges). The model produced stable trajectories with an accuracy close to the DFT reference method while requiring significantly fewer parameters. The Δ-learning scheme was also capable of correctly incorporating long-range interactions within a cutoff of 1.4 nm. The results and findings of the study are likely to enable the use of (QM)ML/MM MD simulations in practical applications, the researchers said.
Reference:
L. Böselt, M. Thürlemann and S. Riniker, Machine Learning in QM/MM Molecular Dynamics Simulations of Condensed-Phase Systems, Journal of Chemical Theory and Computation 17, (5), 2641-2658 (2021). DOI: 10.1021/acs.jctc.0c01112
Low-volume newsletters, targeted to the scientific and industrial communities.
Subscribe to our newsletter