Sonali Parbhoo
Interview by Carey Sargent, EPFL, NCCR MARVEL
The biggest challenge that women scientists face is...
First of all, I feel as if the everyday challenges male and female scientists face in research are not so different. I don’t necessarily like the terms “woman scientist” or “women in science”. We are scientists first. It is uncommon to hear the term “men in science” or “male scientist”. They are simply referred to as scientists. Where women are involved, there is often too much discussion about this as if it is unusual or unlikely that we would enjoy science and perform well at it. In reality, there is not really much of a difference in terms of what we do and what we are capable of doing. Having said that, I am aware that I’ve been fortunate to have grown up in an environment where I was always encouraged to pursue a career in science — in fact, this was considered the norm. In many countries, there are fewer opportunities for girls, and circumstances would then be different. In addition, in many fields, especially computer science, women are still severely underrepresented, and many do not pursue long-term careers in science because of their responsibilities later in life. This is however, changing and I think universities are becoming more aware of this. The University of Basel for instance, has introduced a very successful programme called “antelope” to promote females in science. Such initiatives are a step in the right direction. However, in terms of actual challenges, I don’t see major differences between men and women. At the end of the day, we’re all scientists.
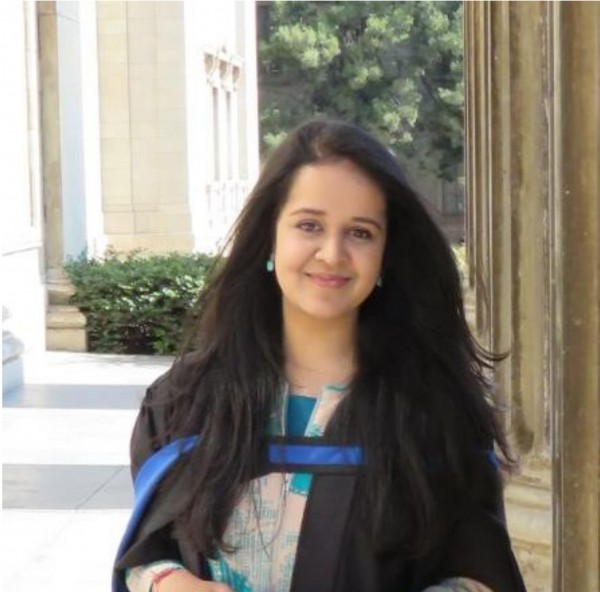
I chose a scientific career because...
As a child, I was genuinely curious about why the world is as it is and would ask a lot of questions. I would say that I was interested in science from the very beginning, and in all scientific subjects. I just didn’t know how I wanted to combine my diverse interests and exactly what I wanted to do. Also, growing up in a Indian family with a strong focus on education, I was always encouraged to pursue a career in science or medicine.
If I weren’t a scientist, I would be…
I don’t know about making a career out of anything else, but I do have a lot of hobbies. I am passionate about writing and in my spare time, I enjoy writing poetry and stories, especially when I travel. I also really enjoy cooking, and at one point recall saying I would like to open my own restaurant. I love playing badminton too, but in reality I don't think I could have made a career out of any of these hobbies. I would however, some day like to be able to use my scientific career to help people and bring about positive change in their lives.
My greatest MARVEL discovery to date…
In all honesty, our group only recently joined the MARVEL project and so I don’t think I’ve made such a big contribution in terms of actual science. Having said that, during my PhD, I’ve developed certain methods for causal modelling and learning interpretable representations of data that can be used for making predictions. Some of these methods can also be applied in the MARVEL project. Currently in our group, we are working on extending some of these ideas to potentially discover new molecules and I think this is very interesting, although it is still work in progress. I will say though that I really enjoy the collaborative aspect of this project. Having been involved in numerous collaborations in the past, some of them more successful than others, the MARVEL project itself particularly excites me because of the vastly different backgrounds of people involved and varying levels of experience.
My top two papers…
In the last maybe four years during my PhD and in machine learning especially, there has been one revolutionary paper that stands out in the ML community and it has really changed the way we think about machine learning and deep learning, which is a big hype at the moment. It’s a paper by Kingma and Welling that talks about variational autoencoders. It describes how traditional generative modelling techniques can be combined with nonparametric deep learning methods to create new models that can not only be used to predict observed things with high accuracy, but can also be used to generate new things. This is important if you think about things like molecules or chemical structures, where you may want to not only determine whether something is a certain compound, but also want to discover how similar it is to other compounds or what would happen to the properties of the molecule and its stability, if certain aspects were changed. Deep generative models allow you to address some of these questions.
Another particularly interesting find in the machine learning community has to be Google’s AlphaGo and AlphaGo Zero papers, where the authors developed the first software ever to beat a human player at the game of Go. Basically the program uses the combination of an algorithm called Monte Carlo Tree Search and deep neural networks to find the best moves to take. While it is not directly related to the MARVEL project, it’s always exciting to see how far along machine learning has come.